Truist Business Research and Innovation Program
2023 Research Grant Awardees
- Online misinformation characterization and detection from social media based on within-post multimodal content consistency
Dongsong Zhang, Belk Endowed Chair Professor, BISOM Department, Belk College of Business
Lina Zhou, Professor, BISOM Department, Belk College of Business
Shi Chen, Associate Professor, Department of Public Health Sciences, CHHS1 Yaorong Ge, Professor, Department of Software and Information Systems, CCI
Daniel Janies, Carol Grotnes Belk Distinguished Professor of Bioinformatics and Genomics/Co-Director for Computational Intelligence to Predict Health and Environmental Risks (Cipher), Department of Bioinformatics, CCI
Health misinformation has infiltrated and proliferated on every social media platform, causing substantial harm to individuals, families, organizations, businesses, and the entire society. The Web 3.0 era further enables misinformation to grow in multimodal social media content, such as images and audio/videos. Within-post inconsistencies across multimodalities, known as content topic and sentiment shift, is an emerging characteristic of misinformation to deceive users and alter their perceptions towards reliable information. Nevertheless, how within-post inconsistencies result in misinformation remains understudied. To keep up with the new trend of multimodal misinformation, we propose a theoretical framework to quantify within-post content inconsistencies across text, image, and audio/video modalities, design a consistency scoring mechanism, and develop a multimodal misinformation detector. This study builds theoretical and technical foundations for future misinformation detection, advance our understanding of multimodal misinformation, and provide much needed preliminary work and empirical evidence.
- Information Frictions and the Efficacy of the PPP Loan in Mitigating the Economic Impact of COVID-19 for Small Businesses
Sungjune Park Professor of MIS, Department of Business Information Systems and Operations Management The Belk College of Business, UNC Charlotte
Chandrasekar Subramaniam Associate Professor of MIS, Department of Business Information Systems and Operations Management The Belk College of Business, UNC Charlotte
We propose to use data from the Small Business Administration and the U.S. Census data to investigate the impact of information frictions on disproportionate disbursements of Paycheck Protection Program (PPP) loans to certain racial and socioeconomic groups. This research contributes to existing literature on the PPP program, the impact of COVID-19 on small businesses, and government subsidy programs by conceptualizing information frictions into three main drivers: socio-demographic bias, financial institution access, and digital literacy. The study is the first of its kind to examine the relationship between information friction and the efficacy of the PPP program.
- Gendered Interactions and Inclusive Leadership (GIIL)*
Dr. Janaki Gooty, Professor, Department of Management; Belk College of Business, University of North Carolina, Charlotte.
Dr. Jill Yavorsky, Assistant Professor, Department of Sociology; College of Liberal Arts and Sciences, University of North Carolina, Charlotte.
Dr. Nicole Strah, Assistant Professor, Department of Management; Belk College of Business, University of North Carolina at Charlotte
Dr. Eric Heggestad, Professor, Department of Psychological Science; College of Liberal Arts and Sciences, University of North Carolina at Charlotte
Leadership has evolved over the last century, especially given the rise of women and people of color in leadership roles. What remains missing in previous leadership models are considerations of how under-represented groups, such as diverse groups of women, may enact behaviors that have not been captured by models derived from racially homogenous groups of men. This project is part of a research program on building inclusive leadership frameworks. Here, we focus on building a taxonomy of leader behaviors that incorporates the behaviors of women leaders, especially, regarding how they modify behaviors (if at all) as a consequence of workplace gendered interactions. We will then build a nomological network of leader behavior comprising inclusive leader behaviors, perceptions of followers, and effectiveness evaluations. Practically, our scholarship will pivot from current leadership training programs that tend to focus on “fixing women,” and instead, turn towards interventions aimed at fixing the system.
- Enhancing leader effectiveness using artificial intelligence.
George Banks, Professor and Chair, Department of Management
Scott Tonidandel, Professor, Department of Management and School of Data Science
The proposed project seeks to develop a novel AI-powered platform to analyze and train leader behavior in the virtual context. The future of work involves a virtual context for many occupations. A new challenge for leadership coaches is to train leaders to perform effectively in a virtual context and to develop a diverse pipeline of leaders. Another challenge for coaches is that there is a need for them to leverage future technology in their training of leaders. We address these challenges by proposing to create a machine learning algorithm that can automatically score charismatic, destructive, ethical, and transformational leader behaviors via meetings transcripts. Working adults will be recruited to participate in virtual meetings. The meetings will be audio recorded and transcribed. Text data will be labeled in Python using Prodigy as an interface. After training, the algorithmic model will be validated by distinct meetings transcripts for training and development purposes.
- A Model For Effective Call Routing to Maximize Customer Satisfaction
Moutaz Khouja, Professor, Business Information Systems and Operations Management Department The Belk College of Business, The University of North Carolina at Charlotte
Customers do not like to wait when they call a service provider nor do they like to make repeated calls about the same issue. Effective call center management is a must for firms that aim for high customer satisfaction. An effective system for call center management has three-components. Component I is a call classification system utilizing machine learning to identify the most important call characteristics for determining the probability of being resolved in the first call (first call/contact resolution). Component II is an agent skill measuring system which ranks agents based on their first call resolution performance for the important call characteristics identified by Component I. Component III, which is the subject of the proposed research, is multi-criteria optimization model (MCOM) to route incoming calls to agents to maximize customer satisfaction. Thus, an incoming call will be classified, i.e., assigned a difficulty index, using component I. For calls with a high difficulty index, the MCOM will be used to assign them to an on-duty agent. The MCOM, using the call characteristics and agent information in Component II, will find a good (and hopefully best) agent to handle a given call. In developing the MCOM, the proposed study will identify the most important system criterion to consider in routing calls and the weight that should be assigned to each criteria. This criterion includes average wait time, average handling time, number of customers in the queue, probability of first call resolution, etc. Both the criterion and the weights will be dynamic, i.e., change with changes in the state of the system. Extensive simulation will be used to decide the best set of criterion and weights for different system states, i.e., number of calls in the queue and number of agents on duty.
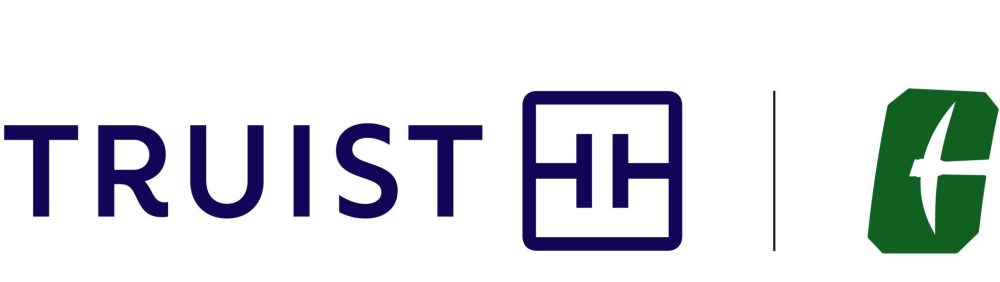